The world is witnessing unprecedented growth in technological advancements, which has led to the emergence of new and innovative tools that are changing the way we live and interact with each other. One of the most exciting developments in this field is data annotation technology, which is driving progress in artificial intelligence and machine learning.
Data annotation is the process of labeling data to make it easier for machines to understand and process. This technology has become increasingly important in recent years as businesses seek to harness the power of AI and machine learning to gain a competitive edge in the market. With data annotation, businesses can train their AI systems to recognize patterns and make predictions based on large amounts of data. This, in turn, allows them to make better decisions and improve their operations.
However, understanding the intricacies of data annotation technology is not always easy. It requires a deep understanding of the different types of data annotation techniques and how they can be applied to different types of data. For example, image annotation involves labeling images with specific tags that describe their content, while text annotation involves labeling text data with relevant information such as sentiment or topic.
To fully leverage the power of data annotation technology, businesses need to be aware of the challenges involved. For instance, data annotation can be time-consuming and expensive, especially when dealing with large datasets. Moreover, the quality of the annotations can vary depending on the expertise of the annotators, which can affect the accuracy of the AI system.
Despite these challenges, the importance and impact of data annotation technology cannot be overstated. It is transforming the way businesses operate by providing them with valuable insights and enabling them to make better decisions. As such, it is important for businesses to keep up with the latest developments in data annotation technology and to invest in the resources necessary to fully leverage its potential.
Table of Contents
What is Data Annotation Tech?
Data annotation technology means the process of labeling or tagging data to make it easier for machines to understand. Its main objective is to promote pattern recognition and understanding of machine learning models. Generating annotated data enables them to use AI in various industries. Facilitates development and improvement of algorithms.
Why is Data Annotation Tech Essential?
1. Enhanced Machine Learning Models: The accuracy and effectiveness of machine learning models heavily depend on the quality of annotated data. Properly labeled datasets enable models to learn and generalize patterns, making them more proficient in tasks like image recognition, natural language processing, and more.
2. Industry Applications: From autonomous vehicles and healthcare to finance and e-commerce, Data Annotation Tech finds applications in diverse industries. It is instrumental in optimizing processes, improving decision-making, and fostering innovation.
3. Training and Validation: Annotated data serves as the foundation for training machine learning models. It allows algorithms to learn from examples, validating their performance and ensuring reliability in real-world scenarios.
4. Semantic Understanding: Data Annotation Tech goes beyond mere labeling; it involves imparting semantic understanding to the data. This means machines not only recognize patterns but also comprehend the context, leading to more accurate and context-aware predictions.
How to Learn Data Annotation?
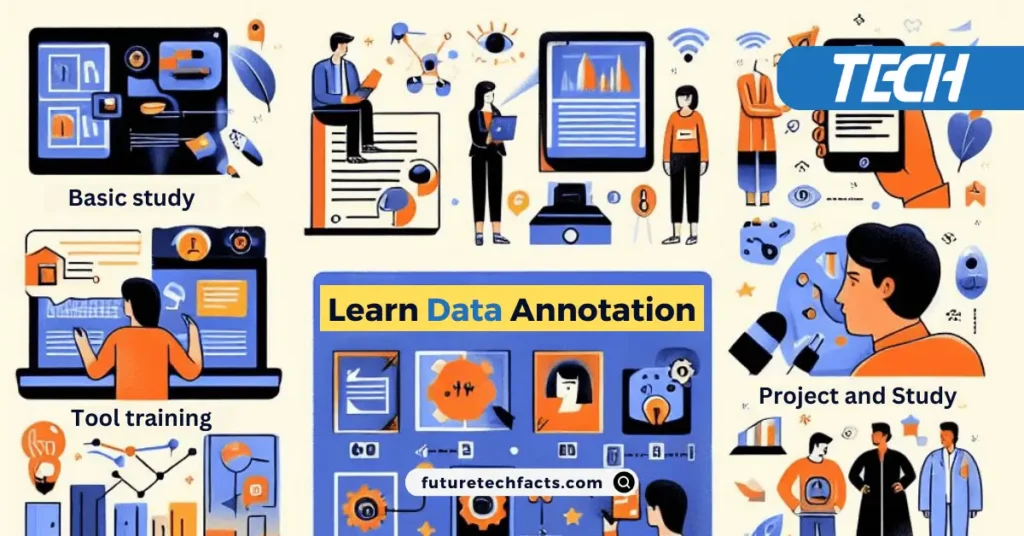
Learning data annotations is an important skill required to train machine learning models. It mainly performs various tasks, such as labeling bounding boxes for images and videos, naming entities for text, etc. Here are some steps you can follow to learn data annotation:
- Basic study: Before getting started with data annotation, you should get a monetary understanding of data annotation. It involves the study of various annotation tasks, tools, and techniques.
- Selecting an annotation tool: You should thoroughly understand which annotation tools are available and what features they offer. Some popular annotation tools are Labelbox, Supervisely, Amazon SageMaker Ground Truth, and VGG Image Annotator (VIA).
- Tool training: When you choose an annotation tool, you’ll need to learn how to use it. Study user manuals, video tutorials, and other resources on the tool’s website.
- Practice with sample data: After learning the content of the tool, practice with sample data. Many annotation tools provide sample data for labeling data so you can hone your skills using the tools.
- Work on different tasks: Work on different annotation tasks according to different data annotation needs. This may include bounding boxes, item classification, Unicode text annotations, and segmentation.
- Work with experienced experts: If possible, working with experienced data annotation experts can give you even better results. By taking their support you can learn new techniques and experiences.
- Precautions and quality control: Follow precautions when annotating data and engage in the quality control process. Include a review process to ensure correct and accurate annotations are important.
- Project and Study: Ensure learning by working and studying on a real project. Draw studies from a variety of sources to boost the process of data annotation to increase exposure.
By following these steps you can learn the art of data annotation and become a competent data annotator who can contribute to training machine learning models.
Recommended to Read:Â What Is Blockchain Technology?
Types of Data Annotation Services:
Data annotation services involve actions that involve labeling or tagging data so that it can provide understanding for machine learning models. These services play a vital role in training and improving artificial intelligence algorithms. Here are some of the main aspects of data annotation services:
Image Annotation:
Annotation of images covers tasks such as object detection, image segmentation, bounding box annotation, and landmark annotation. This is essential for computer vision applications.
Text Annotation:
In natural language processing (NLP) tasks, text annotation services help in labeling objects, sentiment analysis, named entity recognition, and other linguistic components.
Video Annotation:
Video annotation includes tasks such as object tracking, action recognition, and frame annotation. This is important for understanding and understanding the video content.
Audio Annotation:
Audio data annotation includes labeling audio files for tasks such as commercial systems, sentiment analysis, and speaker recognition, enabling effective voice-based AI. Systems develop.
3D Point Cloud Annotation:
For applications such as autonomous vehicles and robotics, 3D point cloud annotation helps in identifying and labeling objects in three-dimensional space.
Lidar Annotation:
Lidar data annotation for autonomous vehicles and similar applications is important to support improved transportation and object detection.
Medical Image Annotation:
In the health sector, medical image annotation is used to help in tumor identification, organ separation, and medical diagnosis.
Geographical Annotation:
Geographic annotation involves labeling location-based data, which contributes to mapping, geotagging, and spatial analysis applications.
Semantic Segmentation:
Semantic segmentation involves labeling each pixel with a corresponding class, allowing AI models to help in understanding and differentiating different objects and areas.
Quality Control and Verification:
Data annotation services incorporate quality control processes to ensure the accuracy and reliability of labeling data. This may involve multiple rounds of annotation, reviews, and verification.
Custom Annotation Services:
Providers often offer annotation services customized to project needs. This ensures that diverse data types and functions can be supported.
Scalability and Efficiency:
Data annotation services are designed to handle large datasets effectively, with the ability to scale based on project requirements. This scalability is important for training models with large and diverse datasets.
Privacy and Security Measures:
A reliable data annotation service ensures the confidentiality and security of private information. It is important to follow data protection regulations and implement secure workflows.
Outsourcing data annotation services to specialist providers can help save time and resources, allowing the organization to focus on developing and improving models. It is important to select a service provider that has expertise in particular annotation tasks related to the project.
Skills Required for a Successful Data Annotator Career:
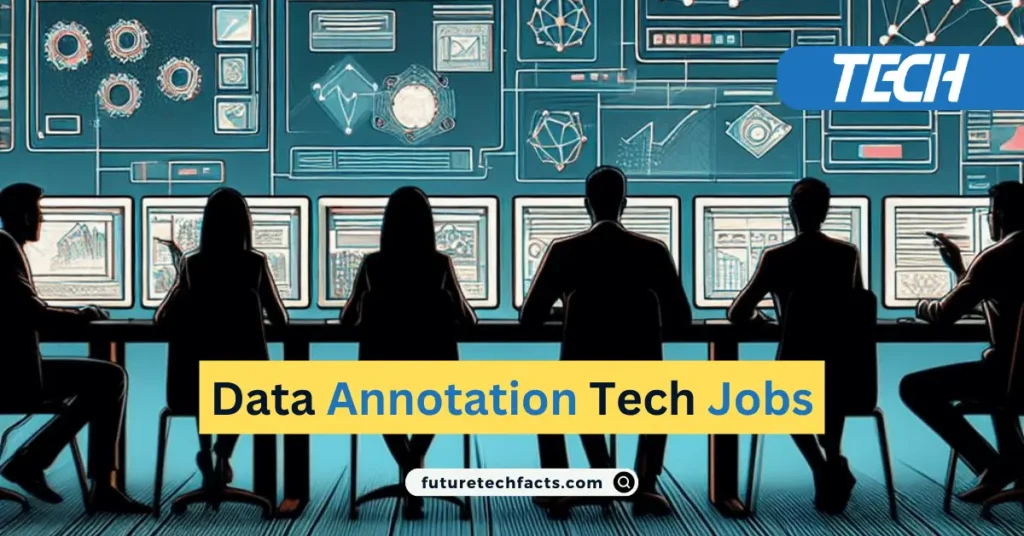
Being proficient as a data annotator requires a specific skill set to ensure accuracy, efficiency, and reliability in the annotation process. The following skills are required to excel in this field:
Data Annotator Job Description:
1. Towards prudence: Indexability is the ultimate in data annotation. Annotators must pay precise attention to the details of each data item to correctly label and classify it.
2. Field Knowledge: As per the basic industry experience, the annotator should have a formative knowledge of the topic. This knowledge helps the annotator’s ability to interpret and label correctly.
3. Familiarity with Annotation Tools: Requires proficiency in using annotation tools and software. Data annotators should be adept at working with different platforms to properly label and tag data according to the project’s needs.
4. Artificial Intelligence: Annotators are often faced with ambiguous or challenging data points. This skill, even in the absence of clear guidelines, is the ability to convince them and make the right decisions.
5. Communication Skills: Clear communication with organization members, project managers, and other relevant entities is extremely important. Annotators need to express their ideas, clarify concepts, and contribute to the conversation to ensure the quality of the annotated data.
6. Compatibility in Annotations: Maintaining consistency in annotations is important to generate reliable datasets. Annotators must follow established guidelines and standards to maintain consistency in labeling.
7. Customization: The field of data annotation is dynamic, with projects frequently evolving. Annotators must have the ability to adapt to project requirements, and new data types, and have excellent annotation techniques.
8. Time Management: Efficiency is required for efficiency in data annotation projects. Annotators must manage their time effectively so that they can maintain accuracy while adhering to deadlines. This involves prioritizing tasks and optimizing workflow.
9. Quality Control: It is important to implement quality control measures, catch errors, and ensure the overall accuracy of the annotated data. Annotators should have the ability to review their work and make necessary corrections.
10. Ethical Circumstances: Data annotators may deal with sensitive information, so must have a strong sense of ethics and privacy. Annotators need to accurately and methodically address the ethical implications of the information being annotated.
By developing and strengthening these skills, individuals can succeed in the dynamic and growing field of data annotation.
Data Annotation Tech Reviews:
Following are some reviews of data annotation tools:
Labelbox Review:
Labelbox is an effective and user-friendly data annotation platform. It includes various annotation tools such as bounding box, polygon, and point cloud. Its security and support are also high.
Supervisely Review:
Supervisely is an open-source annotation platform that includes tools and algorithms for various deep-learning projects. Its good support makes it prominent due to community and self-interest.
VGG Image Annotator (VIA) Review:
VIA is a simple and versatile data annotation tool for annotating images, videos, and linked data. It is designed to be user-friendly and helpful.
Amazon SageMaker Ground Truth Review:
Amazon SageMaker Ground Truth is a full-slate automated annotation service that includes both human and model-based annotations. It is compatible with Amazon Cloud and suitable for large projects.
Prodigy Review:
Prodigy is a secure and user-friendly data annotation tool suitable for research and model development. It has local annotation, text annotation, and integration of external data.
COCO Annotator Review:
COCO Annotator is an open-source data annotation tool designed to annotate textiles, blogs, and items in the desired format.
Hasty.ai Review:
Hasty.ai is an automated data annotation platform that includes algorithms for annotation and is user-friendly. It is suitable for working with large datasets.
Using these reviews you can compare different data annotation tools and select the right tool as per the needs of your project.
Conclusion:
Data annotation technology is an important and functional process that helps support machine learning. Its proper use helps in making safe and high-level decisions in various industries.
Data annotation tech has been the cornerstone of advances in AI and machine learning. Data annotation plays a very important role in refining algorithms and improving machine understanding. The use of the power of AI in various business areas is increasing day by day. A deep understanding of data annotation technology is becoming essential for sustainable growth and innovation in AI. So stay ahead in the technology-driven era by embracing the transformative capabilities of data annotation technology.
FAQ
Q1: What is a data annotation job?
Data annotation work is a process in which human annotators or annotation teams are tasked with adding specific information to a data set. The main objective of this process is to generate accurate and positive data to train machine learning models.
Q2: What is data annotation in machine learning?
Data annotation is an important process in machine learning in which human annotators or annotation teams are tasked with adding specific information to a data set. Its purpose is to provide machine learning models with accurate and consistent data so that they can learn specific tasks. Data annotation can include tasks such as clothing classification, object detection, and text labeling, which help models learn across different data types.
Data annotation in machine learning ensures that models are trained correctly so that they can demonstrate good results. It is important to have a human role in this process as machines need proper knowledge to understand human recognition and train the models correctly.
Q3: What is data labeling and annotation?
Data Labeling:
Data labeling is a process in which each data set instance is classified into specific categories or labels. This is essential for machine learning models to learn so that they can perform specified tasks correctly. For example, categorizing images in a data set into images of different creatures (such as a dog, building, or car) could be an example of data labeling.
Annotation:
Annotation is an annotation that marks data points directly or indirectly as required. This is useful for helping machine learning models learn correctly. Part of the annotation process may be marking particular characteristics of a data set, such as the positions of objects, labeling classifications, or tagging text fragments. The annotation scope can include many different tasks and helps make the data set suitable for models.
Q4: What does a data annotation specialist do?
The job of a data annotation specialist is to help prepare the right data for machine learning models. They are responsible for carefully labeling the data set and adding appropriate annotations for various tasks. The job of these experts is to demonstrate proficiency in various areas, such as object detection, segmentation, or text annotation. Their positive contributions help create high-potential data sets, allowing models to succeed in learning specific tasks.
Q5: How to use data annotation in MVC?
Data annotations in MVC are an important step that helps us ensure that our user experiences are excellent. We can compile data related to users’ favorite views, clothing, and lifestyle through data annotation. This allows us to communicate directly from the user’s perspective. Allows MVC to tailor to better understand user needs and deliver the highest level of new professional services. Thus, data annotation is important to help MVC build a direct relationship with users and understand their needs.